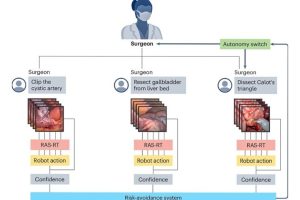
Surgical robots have long been valuable tools in the operating room, assisting surgeons with precision tasks like suturing, holding instruments, or stabilizing organs. However, these systems still depend heavily on direct human control or pre-programmed instructions.
In a perspective piece in Nature Machine Intelligence, a team including Johns Hopkins engineers proposed using multi-modal, general-purpose models—trained on diverse datasets, including video demonstrations—to make surgical robots safer and more efficient. They say this will equip surgical robots with the adaptability and autonomy needed to handle complex, dynamic procedures, reducing the burden on human surgeons.
“One major barrier to achieving fully autonomous surgical robots is the complexity of surgeries themselves,” said lead author Samuel Schmidgall, a graduate research assistant in the Whiting School of Engineering’s Department of Electrical and Computer Engineering. “Surgeons work with soft, living tissue, which changes unpredictably. This makes it hard to simulate real-life conditions in training for surgical robots, and they must operate with high levels of safety to avoid patient harm.” And unlike robots in manufacturing, surgical robots cannot rely solely on trial and error; each step must be meticulously planned to avoid risks.
A single “multi-modal, multi-task” AI training model that combines visual, language, and physical (action-based) data overcomes these challenges, they say.
“This vision-language-action model would allow surgical robots to understand instructions in natural language, interpret what they see in real time, and take appropriate action based on context,” said Schmidgall. “The model could even detect situations that require human intervention, automatically signaling the surgeon when something is unclear.”
Axel Krieger, assistant professor in the Whiting School of Engineering’s Department of Mechanical Engineering and co-author of the paper, recently demonstrated the potential of this approach by training a surgical robot using video-based datasets. Krieger’s work showcases how multi-modal training enables robots to perform intricate tasks autonomously, bridging a gap between theory and practice in surgical robotics.
The emerging multi-modal model used in the Krieger team’s work and discussed in the paper describe the approach’s transformative potential for surgical robotics. By analyzing thousands of procedures, these systems could refine techniques, assist in multiple surgeries simultaneously, and adapt to new scenarios, the authors say, pointing out that such tools could also ease surgeons’ workloads and, ultimately, improve patient outcomes.
Co-authors of the paper also include Ji Woong Kim from the Department of Mechanical Engineering, Johns Hopkins University; Alan Kuntz from the University of Utah; and Ahmed Ezzat Ghazi, Department of Urology, Johns Hopkins Medical Institute.